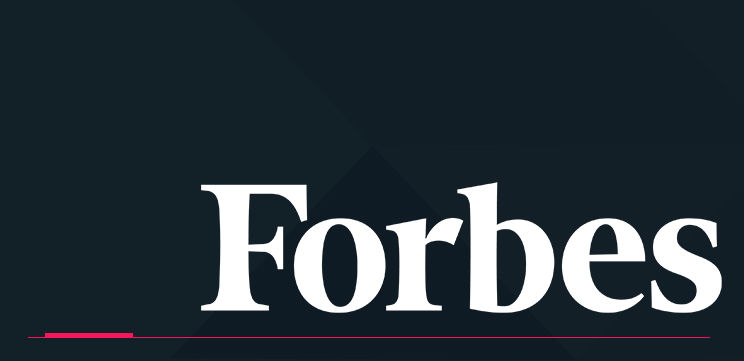
Christopher Rogers is the COO at Carenet Health and has over 20 years of consumer and client engagement experience.
Sentiment analysis, also known as opinion mining, has been a part of the technological landscape for many years. Sentiment analysis leverages natural language processing to evaluate written or spoken language to determine if an emotional tone being expressed is favorable, unfavorable or neutral. By utilizing machine learning, neural networks and other types of artificial intelligence (AI) along with computational linguistics, sentiment analysis can yield insight on how a consumer felt about their customer support experience.
Recently, there’s been a notable surge in the advancement and growth of sentiment analysis, driven by its utilization of large language models and the ongoing refinement of AI technology. In healthcare, for example, there are a range of potential opportunities to elevate the quality of the patient experience and the care they receive through the application of sentiment analysis.
As it evolves and becomes more sophisticated, sentiment analysis platforms can accurately and efficiently gauge a patient’s emotional state and thereby facilitate communication between providers and patients that is more concise and effective. Accordingly, AI-driven emotional recognition tools in healthcare represent an ethically sound application of a technology that can drive better treatment decisions and improve health outcomes.
Leveraging The Value Of Emotional Recognition AI
In the context of virtual care, especially in settings that don’t include video interaction such as phone-based conversations between individuals, clinicians and support staff, leveraging emotional recognition AI could be highly valuable. Without the benefit of nonverbal cues, AI can serve as a supportive cobot by assisting with or conducting repetitive tasks and gauging caller emotions to help inform which processes are working and identifying how improvements could be made in future interactions.
By applying sentiment analysis in this way, healthcare professionals can be presented with clear instructions and tips on how to best adapt their approach in order to optimize how they convey medical advice and deliver care to each individual patient. When leveraged as a training tool with the goal of increasing patient satisfaction and decreasing friction, sentiment analysis can help guide healthcare professionals in effectively navigating virtual engagements based on displayed emotions, comments and symptoms.
Sentiment analysis can identify why an individual was satisfied with the support they received or how they were treated and what is needed to automate and improve future interactions. As a result, people feel that they’re being listened to and cared for, which in turn fosters loyalty and trust.
To provide basic insights into the emotions expressed or detected during support calls, sentiment analysis that doesn’t entail advanced AI-emotion detection is a relatively common component of customer service support centers. Cobots in use today can actively listen to calls and offer recommendations to call center agents for improved outcomes based on learned next steps. When it eventually enters the mainstream, the fusion of these elements to achieve a more profound real-time emotion recognition represents an exciting stage in the evolution of sentiment analysis.
However, the adoption of this technology in healthcare is likely not imminent. It’s almost certain that retail call center agents will incorporate emotional AI cobots before healthcare professionals utilizing virtual care will fully integrate this technology into their workflows. While diagnostic and treatment technologies in healthcare often stand at the forefront of innovation, the implementation of technology for enhancing the patient experience and communication typically lags behind consumer engagement efforts.
The Sensitivity Of Health Data Calls For Safeguards And Regulation
While the ongoing consumerization of healthcare will continue to challenge the industry’s typically slower response than retail’s foray into technology, it’s important to consider the sensitivity surrounding personal health data and health-related conversations that call for strict safeguards.
Despite what the future of AI technology holds, the increasingly rapid pace of AI-driven change has, in a sense, caught the world off guard. As evidenced by the Biden administration’s recent executive order, governments are taking notice that cutting-edge tech needs to be balanced against national security and consumers’ rights.
Although governments at all levels aren’t known for the haste with which they operate, regulation is essential for AI as a whole, and safeguards will need to be developed quickly given the rapidly evolving nature of this technology. Regulation with a special emphasis on generative large language model AI will be critical as existing constraints on generative AI are primarily human-generated. Technologies that unsettle even the most informed experts and business leaders due to their potential require regulation. This will ensure that such tools don’t lead to adverse outcomes, propagate harmful intentions or generate responses based on self-learned information, rendering the tool either ineffective or risky.
Nevertheless, by recognizing the risks involved and taking steps to guard against how the technology is used, sentiment analysis can play a critical role in enhancing the patient experience. As healthcare strives to keep pace technologically with industries like retail and finance, it will be compelling to gauge the evolution of sentiment analysis and its potential to foster more meaningful connections between patients and providers.